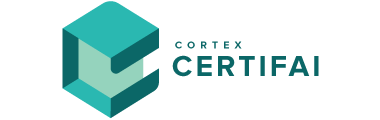
Cortex Certifai
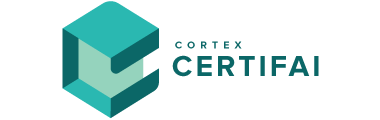
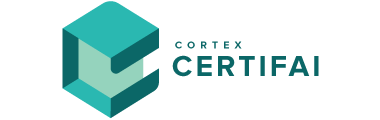
Cortex Certifai
By CognitiveScale Inc
Certified enterprise ready
Gain visibility and control over automated decisions by managing the risk and optimizing the value of black-box AI models. Certifai automatically detects and scores model risks including performance, data drift, bias, explainability, and robustness.
Software version
1.3
Runs on
OpenShift 4.6 - 4.9
Delivery method
Operator
Products purchased on Red Hat Marketplace are supported by the provider. Beyond documentation and developer communities, specialists and product maintainers may be available to address your concerns.
FAQs
Fairness is one of the output reports optional for each project in Certifai. Certifai projects analyze one or more models using the same or comparable datasets.
Fairness is a measure of the outcome disparity between categorical groups defined by the selected dataset feature.
Fairness is a particular concern in AI systems because bias exhibited by predictive models can render models untrustworthy and unfair to one or more target groups.
For example, different models can exhibit any number of biases towards features like gender, age, or educational level.
Example: In the use case of binary classification models that are predicting if a loan applicant will be granted or denied a loan, Certifai users might want to decide which model shows a higher level of fairness between male, female, and self-assigning applicants. In this case the data feature, "sex" is identified as the target feature and each model is assigned a burden score that assesses the model's fairness.
Robustness is one of the primary output visualization provided for projects in Certifai. Certifai projects analyze one or more models using the same or comparable datasets.
The NCER Score is the Normalized Counterfactual Explanation-based Robustness Score.
Robustness is a measure of how well a model retains a specific outcome given small changes in data feature values.
Changes to data and, therefore, robustness is of particular concern in AI systems because data changes can be introduced in two harmful ways: maliciously, in the form of data breaches or users gaming the system, and unintentionally when prediction inputs diverge from training data.
A robust model will tend to result in the same prediction regardless of small changes in the input values.
Explainability measures the average simplicity of the counterfactual explanations provided for each model.
The Explanations report provides a record by record view of the actual input data values side-by-side with the counterfactual values, so viewers can observe the suggested amount of change required to move from one outcome to another. The fewer feature values that must be changed to change the outcome of each records, the more explainable the model is.
The explanations report may be run on the entire dataset used to score robustness and fairness or more typically on another dataset the models can accept. This report must analyze a great deal of data, so it may take some time to run for larger datasets.